How does Retrieval Augmented Generation work?
In a nutshell, Retrieval Augmented Generation (RAG) optimizes the outputs of Large Language Models (LLMs) by cross-referencing external knowledge bases. This is done without the need for retraining these models, which makes RAG a cost-effective and efficient approach to enhancing AI solutions. The process involves utilizing semantic search technologies to scan vast libraries of information and retrieve data that is relevant to the specific query at hand.
The integration of this external data enables LLMs to generate more precise and contextually accurate responses. This not only improves user trust in AI applications but also ensures they are receiving up-to-date information. Companies such as Amazon provide managed services like Bedrock and Kendra that simplify the implementation of RAG, making it easier for developers to improve their generative AI applications.
RAG combines traditional information retrieval systems with LLMs in a way that tailors accurate and current information according to specific needs. By leveraging advanced search engines and multimodal embeddings, RAG ensures that only the most pertinent facts are retrieved - improving both relevance and quality in generated text.
Benefits of using Retrieval Augmented Generation
One of the standout benefits of Retrieval Augmented Generation (RAG) is its ability to optimize Large Language Models (LLMs) without the need for retraining. This makes it a cost-effective solution for enhancing AI applications. By referencing external authoritative knowledge bases, RAG improves both the accuracy and relevance of responses generated by these models.
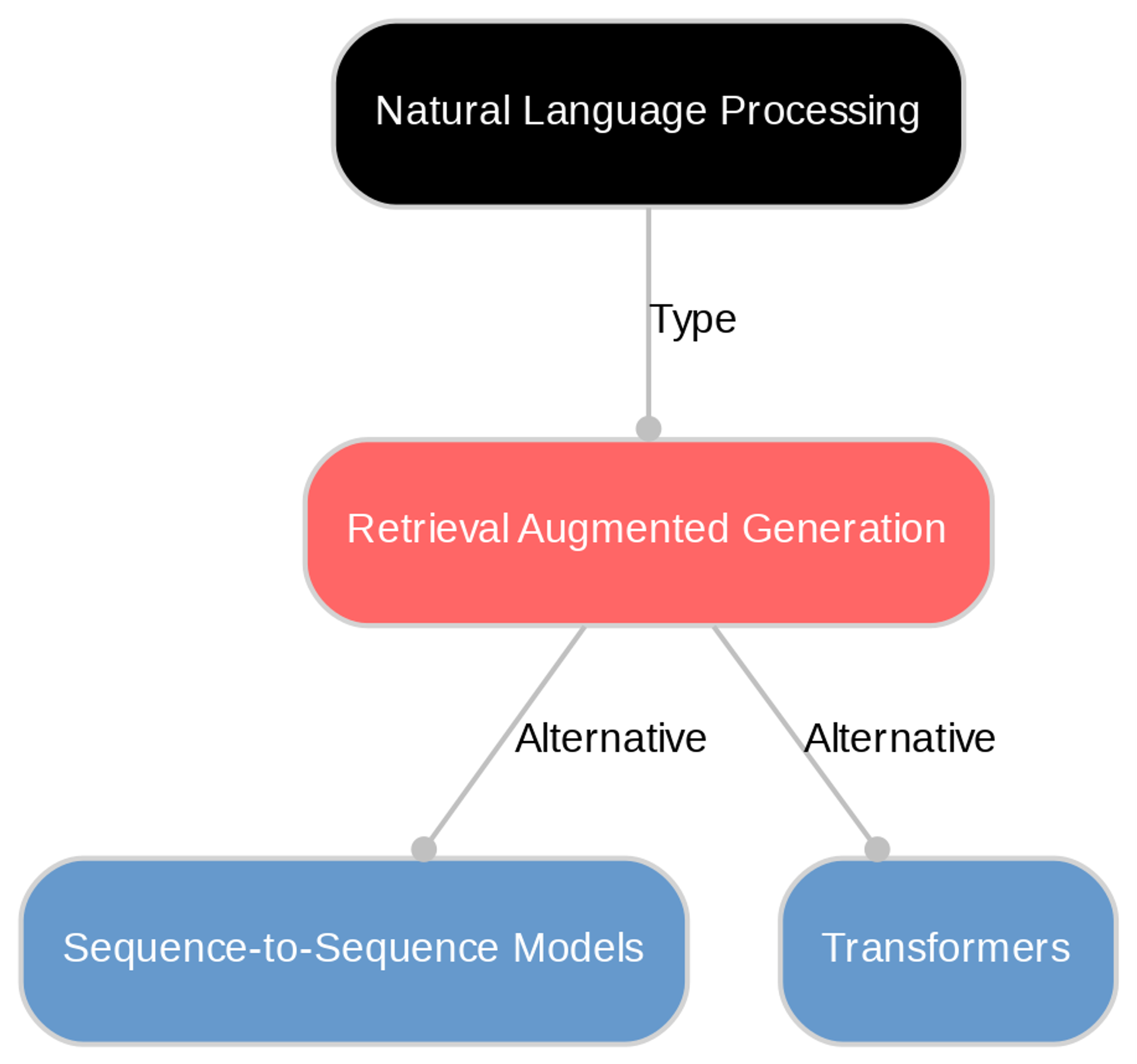
In addition to upgrading response quality, RAG also bolsters user trust by attributing sources and presenting up-to-date information. This transparency in source citation fosters confidence in generative AI solutions, a factor that is crucial for user retention and satisfaction.
RAG's capacity to access specific domains or organizational knowledge further enhances its utility. It enables developers to create more contextually relevant generative AI applications - be it chatbots or other interactive platforms.
Practical applications of Retrieval Augmented Generation
Retrieval Augmented Generation (RAG) finds its practical use in various generative AI applications, significantly enhancing their accuracy and performance. It proves to be a game-changer for chatbots and other interactive platforms that rely on LLMs for providing responses. By using RAG, these applications can generate more reliable and personalized outputs based on the user's query.
In addition to this, RAG aids in reducing inaccuracies in AI responses by retrieving information from live data sources. This function makes it an ideal tool for organizations looking to control the quality of their generative AI solutions while also keeping them up-to-date with relevant information.
Discover More with Sanity
Understanding Retrieval Augmented Generation is just the beginning. Take the next step and discover how Sanity can enhance your content management and delivery.
Last updated: